Introduction: In today's data-driven world, the roles of data analysts and data scientists are often used interchangeably, leading to confusion regarding their actual responsibilities and skill sets. While both roles are crucial in harnessing the power of data, they have distinct differences in terms of their focus, skills, and responsibilities. In this article, we will explore the key similarities and differences between data analysts and data scientists, shedding light on the unique contributions each role makes to the world of data science.
Key Similarities:
Data Handling: Both data analysts and data scientists work extensively with data. They collect, clean, and preprocess data to make it suitable for analysis. This involves handling data in various formats, such as structured and unstructured, and often dealing with large datasets.
Data Visualization: Data analysts and data scientists use data visualization tools to present their findings in a comprehensible and visually appealing manner. This includes creating charts, graphs, and dashboards to help stakeholders understand the insights derived from the data.
Statistical Analysis: Statistical analysis is a common thread between these roles. Both data analysts and data scientists use statistical techniques to uncover patterns, relationships, and trends within the data. They may employ descriptive statistics, hypothesis testing, and regression analysis, among other methods.
Domain Knowledge: A fundamental requirement for both roles is a strong understanding of the specific domain or industry they work in. This knowledge helps them ask relevant questions, interpret results, and make informed recommendations based on data-driven insights.
Key Differences:
Focus and Objectives: The primary distinction between data analysts and data scientists lies in their focus and objectives. Data analysts typically focus on descriptive analytics, where they examine historical data to answer specific business questions. In contrast, data scientists delve into predictive and prescriptive analytics, aiming to build models that can make future predictions and optimize decision-making.
Skill Set: Data scientists are expected to have a more extensive skill set than data analysts. Data scientists are proficient in programming languages such as Python and R, and they possess a strong background in machine learning, deep learning, and artificial intelligence. Data analysts, on the other hand, may not require advanced programming skills and typically use tools like Excel, SQL, and data visualization software.
Data Complexity: Data scientists often deal with more complex and unstructured data. They are skilled in handling big data, working with unstructured data sources like text, and creating machine learning models. Data analysts typically work with structured data and focus on generating reports and dashboards to inform business decisions.
Problem-Solving: While both roles involve problem-solving, data scientists are typically tasked with solving more complex and open-ended problems. They design and implement algorithms to predict future outcomes and optimize processes. Data analysts solve more specific, predefined problems using historical data to guide decisions.
Decision-Making: Data analysts support day-to-day decision-making by providing historical insights and monitoring key performance indicators. Data scientists, on the other hand, often work on strategic projects that require long-term planning and have a substantial impact on the organization's direction.
Conclusion: Data analysts and data scientists are both integral to the field of data science, yet they have distinct roles and responsibilities. Data analysts focus on descriptive analytics, using historical data to answer specific questions and provide insights for day-to-day decision-making. Data scientists, on the other hand, engage in predictive and prescriptive analytics, leveraging more advanced tools and techniques to address complex, future-oriented challenges.
Organizations often benefit from having both roles in their data teams, as data analysts can provide the necessary groundwork for data scientists to build upon. Ultimately, understanding the similarities and differences between these roles is essential for organizations to make informed decisions about staffing and to maximize the value of their data.
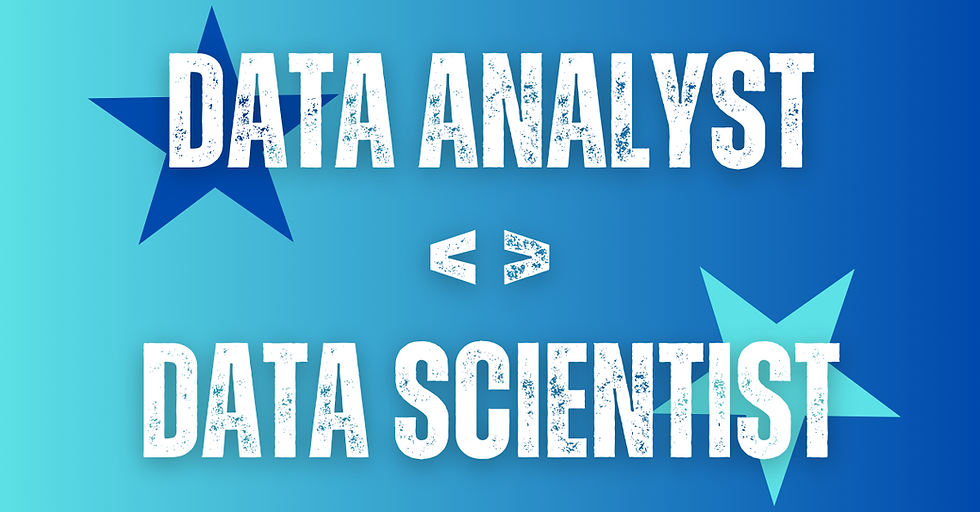
Comments